Do distinguish the difference between an actual cause and effect relation and a simple correlation (without a cause and effect). To do so, think about a possibility of (1) just a coincidence, (2) a relationship based on another (or other) variable(s), and (3) an inverse cause and effect (cause and effect are the other way around).
(2) A confounding factor could affect both (seeming) cause and effect simultaneously; in that case, the deceptive cause and effect actually do not have the relationship.
To avoid (1), (2), and (3), counterfactual thinking is an important approach although a counter-fact cannot be observed; it has to be estimated.
The Financial Journal is a blog for all financial industry professionals. This blog has been, and always will be, interactive, intellectually stimulating, and open platform for all readers.
AdSense
Subscribe to:
Post Comments (Atom)
Deep Learning (Regression, Multiple Features/Explanatory Variables, Supervised Learning): Impelementation and Showing Biases and Weights
Deep Learning (Regression, Multiple Features/Explanatory Variables, Supervised Learning): Impelementation and Showing Biases and Weights ...
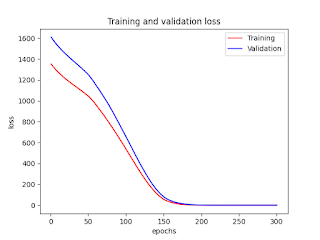
-
This is a great paper to understand having and applying principles to day-to-day business and personal lives. If you do not have your own ...
-
0_MacOS_Python_setup_for_Quandl.txt # Go to: https://www.quandl.com/ # Sign up / in with your email address and password # Run Termina...
-
MSCI INTEGRATED FACTOR CROWDING MODEL Assessing Crowding Risks in Equity Factor Strategies https://www.msci.com/documents /10199/acf506d5-4...
No comments:
Post a Comment