Black-Litterman Portfolio Optimization with Python
This is a very basic introduction of the Black-Litterman portfolio optimization with the Python coding samples.
[0] Traditional Optimization: Mean-Variance Approach by Markowitz
In the mean-variance approach, we have to estimate both expected returns and variance-covariance (risks), and then optimize our portfolios by maximizing its return and minimizing its risk (variance or standard deviation, i.e., volatility, of the return). This is a very straightforward approach, but there are some practical issues:
Risks are relatively stable and easier to estimate while returns are unstable and harder to estimate
You have to estimate returns of every single asset in the mean-variance approach; also, if the expected returns vary, the optimization result (optimized portfolio) changes a lot.
[1] Reverse Optimization and Bayesian Inference: Black-Litterman Approach
In the Black-Letterman approach, expected returns are not directly estimated; instead, it hypothesizes that the entire market itself is optimally allocated. Namely, the current market portfolio with market cap weights are derived based on the market-estimated risks and returns. It calculates expected (or implied) returns by using the current market cap weights and estimated risks.
Further more, an investor’s view is blended into the estimated equilibrium returns above by using the Bayesian inference. For instance, an investor’s view goes like this. An asset A outperforms an asset B by 5%, or an asset C’s return is 5%. For each view, an investor can input confidence as a parameter. An confident view for a return has a bigger impact on the expected portfolio return.
Finally, based on updated expected returns (=implied returns + views and confidence) and risks, an optimal portfolio is computed.
[2] Black-Letterman Portfolio Optimization with Python
# Intro
#
# On the Mac OS, run Terminal and then use the following “python” command before running the following Python scripts:
#
# python
# Let’s reproduce the results in the following paper:
# He & Litterman (1999)
# https://faculty.fuqua.duke.edu/~charvey/Teaching/IntesaBci_2001/GS_The_intuition_behind.pdf
import numpy as np
import pandas as pd
import matplotlib.pyplot as plt
# Equilibrium Portfolio Weights
# p.16 Appendix A Table 1
# AUL, CAN, FRA, GER, JAP, UKG, USA
w = np.array([[0.016, 0.022, 0.052, 0.055, 0.116, 0.124, 0.615]]).T
# Correlation Matrix
# p.16 Appendix A Table 2
# AUL, CAN, FRA, GER, JAP, UKG, USA
correlation = np.array([
[1, 0.488, 0.478, 0.515, 0.439, 0.512, 0.491],
[0.488, 1, 0.664, 0.655, 0.310, 0.608, 0.779],
[0.478, 0.664, 1, 0.861, 0.355, 0.783, 0.668],
[0.515, 0.655, 0.861, 1, 0.354, 0.777, 0.653],
[0.439, 0.310, 0.355, 0.354, 1, 0.405, 0.306],
[0.512, 0.608, 0.783, 0.777, 0.405, 1, 0.652],
[0.491, 0.779, 0.668, 0.653, 0.306, 0.652, 1]])
# Standard Deviation (Volatility)
# p.16 Appendix A Table 2
# AUL, CAN, FRA, GER, JAP, UKG, USA
std = np.array([[0.16, 0.203, 0.248, 0.271, 0.21, 0.2, 0.187]])
# Variance Covariance Matrix (which can be calculated with the correlation and volatility above)
Sigma = correlation * np.dot(std.T, std)
# delta (δ): risk aversion parameter (scalar)
# p.4 footnote
delta = 2.5
# tau (τ): a scalar measures the uncertainty of the CAPM prior
tau = 0.05
### (1) Reverse Optimization
### Inputs: Equilibrium Portfolio Weights, Standard Deviation (Volatility)
### Output: Equilibrium Expected Returns (p.16 Appendix A Table 1)
#
# U: the utility function of the optimization - w which maximizes U is a set of weights for the optimized portfolio
# U= wTΠ − δ/2wTΣw
#
# When w maximizing U, the following equation must hold:
# dU/dw = Π − δΣw = 0
# Π = δΣw
#
# Π: Equilibrium Expected Returns (nx1 vector) (p.16 Appendix A Table 1) —> r_eq
# Π′: Black-Litterman expected returns updated by an investor’s view (nx1 vector) (p.7 Chart 2A) —> r_posterior
# w: Equilibrium Portfolio Weights (nx1 vector) (p.16 Appendix A Table 1)
# w′: Optimal Portfolio Weights (nx1 vector) (p.9 Chart 3B)
# Σ: Variance Covariance Matrix
# P, Q: Investor’s view matrix
# Ω: Investor’s view confidence matrix
# delta (δ): investor’s risk aversion parameter (scalar)
# tau (τ): a scalar measures the uncertainty of the CAPM prior, variance-covariance matrix
#
# reverse optimization
r_eq = delta * np.dot(Sigma, w)
# >>> r_eq
#array([[ 0.03937555],
# [ 0.0691519 ],
# [ 0.08358087],
# [ 0.0902724 ],
# [ 0.0430281 ],
# [ 0.06767693],
# [ 0.07560047]])
### (2) Blending Equilibrium Portfolio Weights with Investor’s Views
### Inputs: Π Equilibrium Expected Returns, P & Q Investor’s view matrix
### Output: Π′ Black-Litterman expected returns updated by an investor’s view —> r_posterior, Σ′ —> Sigma_posterior
#
# Investor’s View
# AUL, CAN, FRA, GER, JAP, UKG, USA
#
# P = [0 0 -0.295 1 0 -0.705 0
# 0 1 0 0 0 0 -1]
# In the first row,
# -0.295: FRA
# 1:GER
# -0.705: UKG
# In the second row,
# 1: CAN
# -1: USA
#
# Q = [ 0.05
# 0.03]
#
# These mean that:
# GER outperforms FRA and UKG by 5%
# CAN outperforms USA by 3%
#
#
# Ω: Investor’s view confidence matrix
# Ω = [ 0.001065 0
# 0 0.000852 ]
P = np.array([
[0,0,-0.295,1,0,-0.705,0],
[0,1,0,0,0,0,-1]]) # 2x7 matrix (2: number of views, 7: number of assets)
Q = np.array([[0.05],[0.03]]) # 2-vector
Omega = np.array([
[0.001065383332,0],
[0,0.0008517381]])
# Black-Litterman master equation
# Π′ = Π + τΣPT (PτΣPT + Ω)-1 (Q−PΠ)
#
# Blending Investor’s View with the Equilibrium Returns
r_posterior = r_eq + np.dot( np.dot( tau*np.dot(Sigma,P.T), np.linalg.inv(tau*np.dot(np.dot(P,Sigma),P.T)+Omega)), (Q-np.dot(P,r_eq)))
#
# AUL, CAN, FRA, GER, JAP, UKG, USA
#
# >>> r_posterior
#array([[ 0.04422145],
# [ 0.08729864],
# [ 0.09479745],
# [ 0.11209947],
# [ 0.04616347],
# [ 0.0697166 ],
# [ 0.0748156 ]])
#
#
# On top of the returns, variance-covariance matrix can be updated as follows:
# Σ′ = Σ + τΣ -τΣPT (PτΣPT + Ω)-1PτΣ
Sigma_posterior = Sigma + tau*Sigma - tau*np.dot( np.dot( np.dot(Sigma,P.T), np.linalg.inv(tau*np.dot(np.dot(P,Sigma),P.T)+Omega)), tau*np.dot(P,Sigma))
#
#>>> Sigma_posterior
#array([[ 0.02684723, 0.01657429, 0.0198378 , 0.02328236, 0.01547005,
# 0.01718801, 0.01539182],
# [ 0.01657429, 0.04299381, 0.03494199, 0.03753285, 0.01383059,
# 0.02589072, 0.03107504],
# [ 0.0198378 , 0.03494199, 0.06439533, 0.06036839, 0.01937078,
# 0.04074256, 0.03244592],
# [ 0.02328236, 0.03753285, 0.06036839, 0.07627367, 0.02106627,
# 0.04414171, 0.03454918],
# [ 0.01547005, 0.01383059, 0.01937078, 0.02106627, 0.04629476,
# 0.01785243, 0.01260504],
# [ 0.01718801, 0.02589072, 0.04074256, 0.04414171, 0.01785243,
# 0.04199287, 0.0255861 ],
# [ 0.01539182, 0.03107504, 0.03244592, 0.03454918, 0.01260504,
# 0.0255861 , 0.03661572]])
### (3) Optimization - finding optimal weights
### Inputs: Π′, Σ′
### Output: w′ Optimal Portfolio Weights —> w_posterior
#
# w′ = Π′ (δΣ′)-1
#
# Forward Optimization and finding optimal weghts
w_posterior = np.dot(np.linalg.inv(delta*Sigma_posterior), r_posterior)
#
# AUL, CAN, FRA, GER, JAP, UKG, USA
# >>> w_posterior
#array([[ 0.0152381 ],
# [ 0.41863571],
# [-0.03409321],
# [ 0.33582847],
# [ 0.11047619],
# [-0.08173526],
# [ 0.18803095]])
#
# Plot w′ by using pandas
df = pd.DataFrame([w.reshape(7),w_posterior.reshape(7)],
columns=['AUL','CAN','FRA','GER','JAP','UKG','USA'],
index=['Equilibrium Weights','Constrained Optimal Weights'])
df.T.plot(kind='bar', color='br')
The Financial Journal is a blog for all financial industry professionals. This blog has been, and always will be, interactive, intellectually stimulating, and open platform for all readers.
AdSense
Subscribe to:
Post Comments (Atom)
Deep Learning (Regression, Multiple Features/Explanatory Variables, Supervised Learning): Impelementation and Showing Biases and Weights
Deep Learning (Regression, Multiple Features/Explanatory Variables, Supervised Learning): Impelementation and Showing Biases and Weights ...
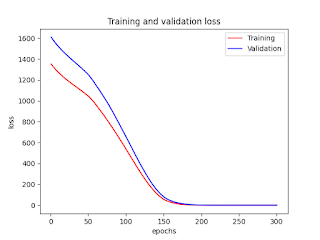
-
0_MacOS_Python_setup_for_Quandl.txt # Go to: https://www.quandl.com/ # Sign up / in with your email address and password # Run Termina...
-
Black-Litterman Portfolio Optimization with Python This is a very basic introduction of the Black-Litterman portfolio optimization with t...
-
MSCI INTEGRATED FACTOR CROWDING MODEL Assessing Crowding Risks in Equity Factor Strategies https://www.msci.com/documents /10199/acf506d5-4...
Hi, you may want to slightly change the way you transform you correlation in covariances. An example could be
ReplyDeletediag = np.diag(std)
Sigma = np.dot(np.dot(diag,correlation),diag)
Portfolio Optimization ist the most import tool for portfolio management. You will find a great job in Portfolio Management
ReplyDelete